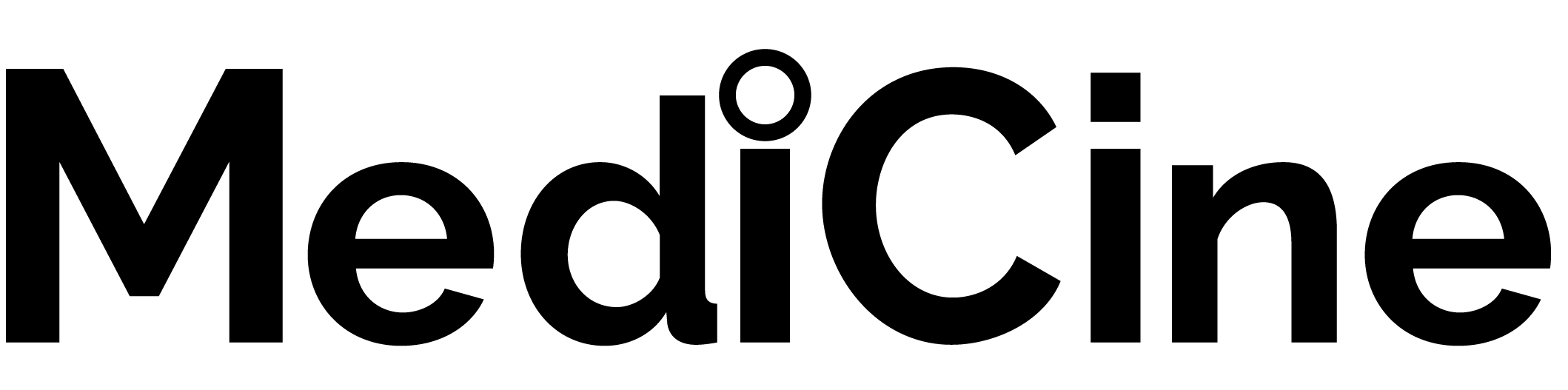
Issue 48
Nov 2023
ETHICALLY SPEAKING
By Dr Sebastian Porsdam Mann, University of Oxford and Professor Julian Savulescu, Director, Centre for Biomedical Ethics, YONG LOO LIN SCHOOL OF MEDICINE, NATIONAL UNIVERSITY OF SINGAPORE (NUS MEDICINE)
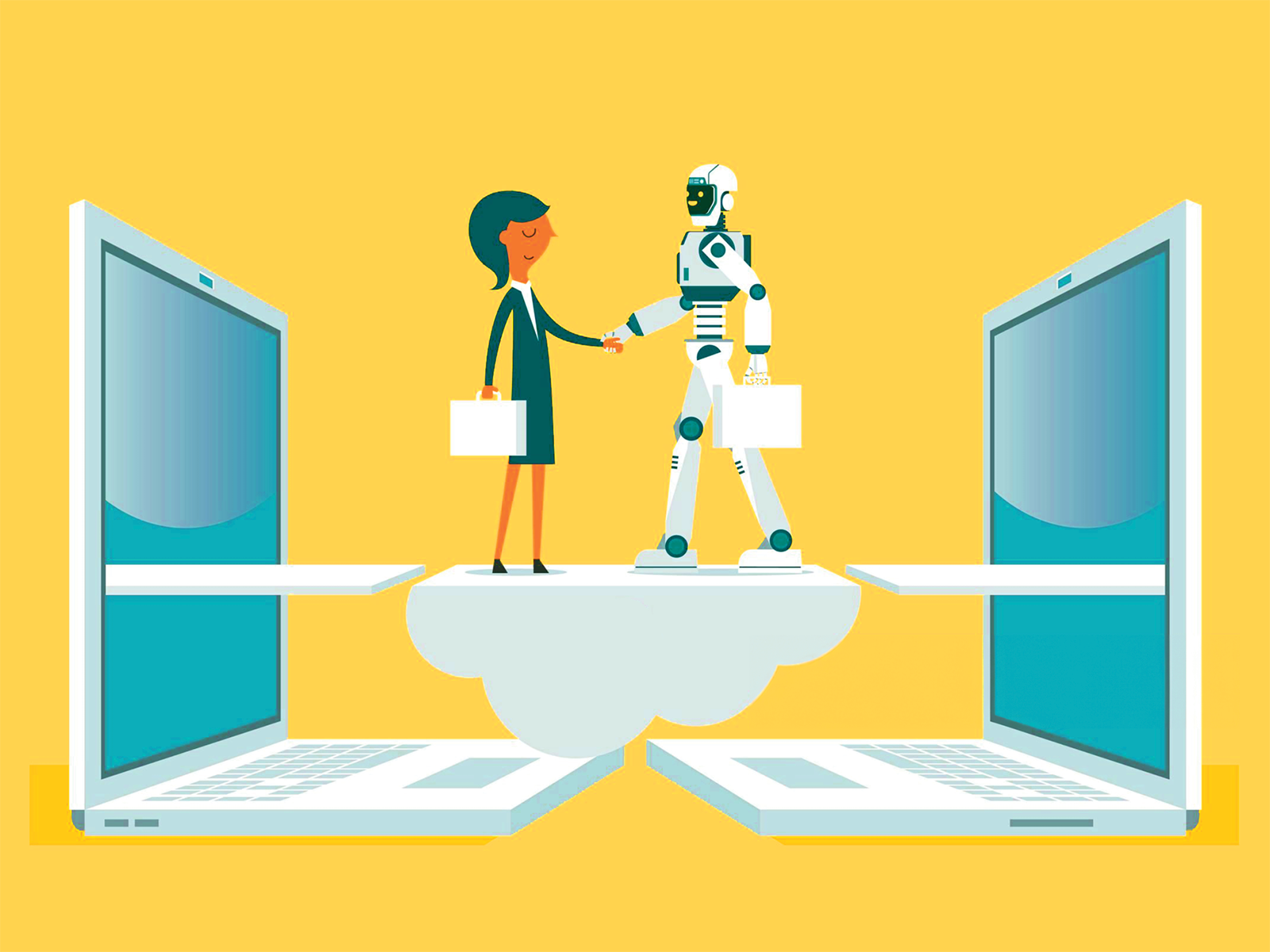
In Goethe's "The Sorcerer's Apprentice", an eager apprentice, left alone, uses magic to avoid chores by making a broom fetch water for him. The unintended results—multiplication of brooms, rampant flooding, desperate attempts to stop the mad magic—are familiar to most of us, thanks to Disney’s beautiful rendition of this cautionary tale (Fantasia).
In an age where the powers of Artificial Intelligence (AI) models have advanced to a point comparable to the apprentice’s magic, the story offers a poignant warning of what can happen when we set in motion powerful forces without sufficient skill or foresight.
One means and source of such foresight is ethical analysis of AI uses. Like many other academic areas, practical ethics has been galvanised by the release of generative AI models such as ChatGPT (a large language model or ‘LLM’) or MidJourney (a text-to-image generator), which allow users to generate words, sounds, or images in near-real time from natural language descriptions. These instructions, were they not written down, would seem to resemble nothing so much as spells and incantations. Yet in real life, we do not have the luxury of waiting for the return of the sorcerer to undo our mistakes and mop up the mess. It is therefore even more important for us than for the sorcerer’s apprentice to foresee and forestall potential problems and pitfalls before releasing our brooms into the wild.
Ethics and LLMs
LLMs are machine learning programs that have learned patterns and associations in huge amounts of natural language sourced from the internet. They work by predicting the statistically most likely next word (or fragment of a word), based on all the preceding text. Although undoubtedly very powerful and useful, these models have also resulted in numerous warnings being sounded. These include concerns based on the (lack of) equal representation in the text used as training data—which comes disproportionately from young, English- and Chinese-speaking men. Among other well-known concerns are the fact that these models cannot yet distinguish reliably between falsehood and truth in all cases and therefore often make up (‘hallucinate’) information. Another concern is the environmental impact of massive amounts of computation expended to train and operate these models.
In our work as practical ethicists focused on biomedical issues—bioethicists—these are familiar concerns whenever a novel, powerful technology comes around. However, there are also fascinating ethical issues unique to generative AI programs such as LLMs which have no parallels to other technologies. Convinced of the importance of understanding issues unique to this powerful technology, we set out to investigate these in a series of papers co-authored with colleagues from across the physical and academic world.
One set of issues concerns who can take credit and who must shoulder blame for harms and benefits arising from LLM use. Like the sorcerer’s apprentice, a LLM user attempts to formulate a set of instructions which sets in motion an autonomous process, the results of which will affect the interests and well-being of others.
Credit and blame
One set of issues concerns who can take credit and who must shoulder blame for harms and benefits arising from LLM use. Like the sorcerer’s apprentice, a LLM user attempts to formulate a set of instructions which sets in motion an autonomous process, the results of which will affect the interests and well-being of others. Intuitively, we know who is to blame in Goethe’s story: the apprentice is reckless or at least negligent with respect to the risks and bites off a larger piece of magical pie than can be digested. Similarly, we hypothesised that users of LLMs are also fully responsible for harms resulting from their use of these programs, even where these were not intended.
In a recent paper in Nature Machine Intelligence,1 we argued that the reverse situation does not hold: where LLM use results in benefits, users cannot take full credit for these. Had the sorcerer’s apprentice been lucky and cast a spell better fit for purpose, they would not deserve as much credit for cleaning up the sorcerer’s lair as they would had they done so on their own. This is because, as colleagues and one of us has previously argued,2 to deserve credit one must have made sacrifices, used skills, or at least employed effort—elements which are notably missing in cases where LLMs (or, for that matter, other humans) are instructed to carry out one’s work.
Based on this insight, we argued that LLM use entails a credit-blame asymmetry: “the use of generative AI elevates the bar for earning credit, but standards for assigning blame remain the same.” If this is the case, this insight has numerous implications for how we should approach the ethical evaluation of LLM use in contexts such as work and education. One important consequence may be what we call the achievement gap: “good, useful outcomes will be produced, but many of them will not be achievements for which human workers and professionals can claim credit.”
Empirical work confirms the existence of a credit-blame asymmetry
In as-of-yet unpublished experimental work, we surveyed samples in the UK, US, Singapore, and China, and found that respondents do indeed assign similar levels of blame, but much reduced amounts of credit for LLM users, as compared to completing the same work without help from an LLM. Interestingly, however, we found no differences across surveys between credit given for text written without LLMs and text written using personalised LLMs. A personalised LLM, as we use the concept, is a language model adapted to information pertaining to a specific individual.
The ethical implications of this are numerous: on the positive side, increased productivity and potential for generating new ideas which can stimulate scientific progress; on the negative side, style theft and impersonation as well as multiple other potential misuses.
Personalising LLMs
We first introduced the concept of personalised LLMs in the specific context of academic prose generation.3 As noted above, LLMs in general are trained on a vast trove of mostly indiscriminately collated internet text. For this reason, LLMs are jacks-of-all-trades, yet master of none: models trained on more specific, specialised datasets outperform, as a rule, more general models. One way to combine elements of both general and special-purpose models is known as fine-tuning and involves exposing an existing, general LLM (such as GPT-3, the precursor to ChatGPT) to a more specialised dataset. The result is a model based on a general purpose LLM, but adapted to a more specific context: a jack-of-all-trades with further training in one of those trades.
One way to personalise an LLM is thus to fine-tune it on material relevant to or produced by an individual. To try this out, we created a custom dataset consisting of the previously published academic articles of ourselves and another of our collaborators (Brian Earp) and fine-tuned GPT-3 on this custom dataset. The result was striking: the fine-tuned model appeared to adapt to our style of argumentation, producing much more coherent and detailed responses to our queries in the style of an academic paper.
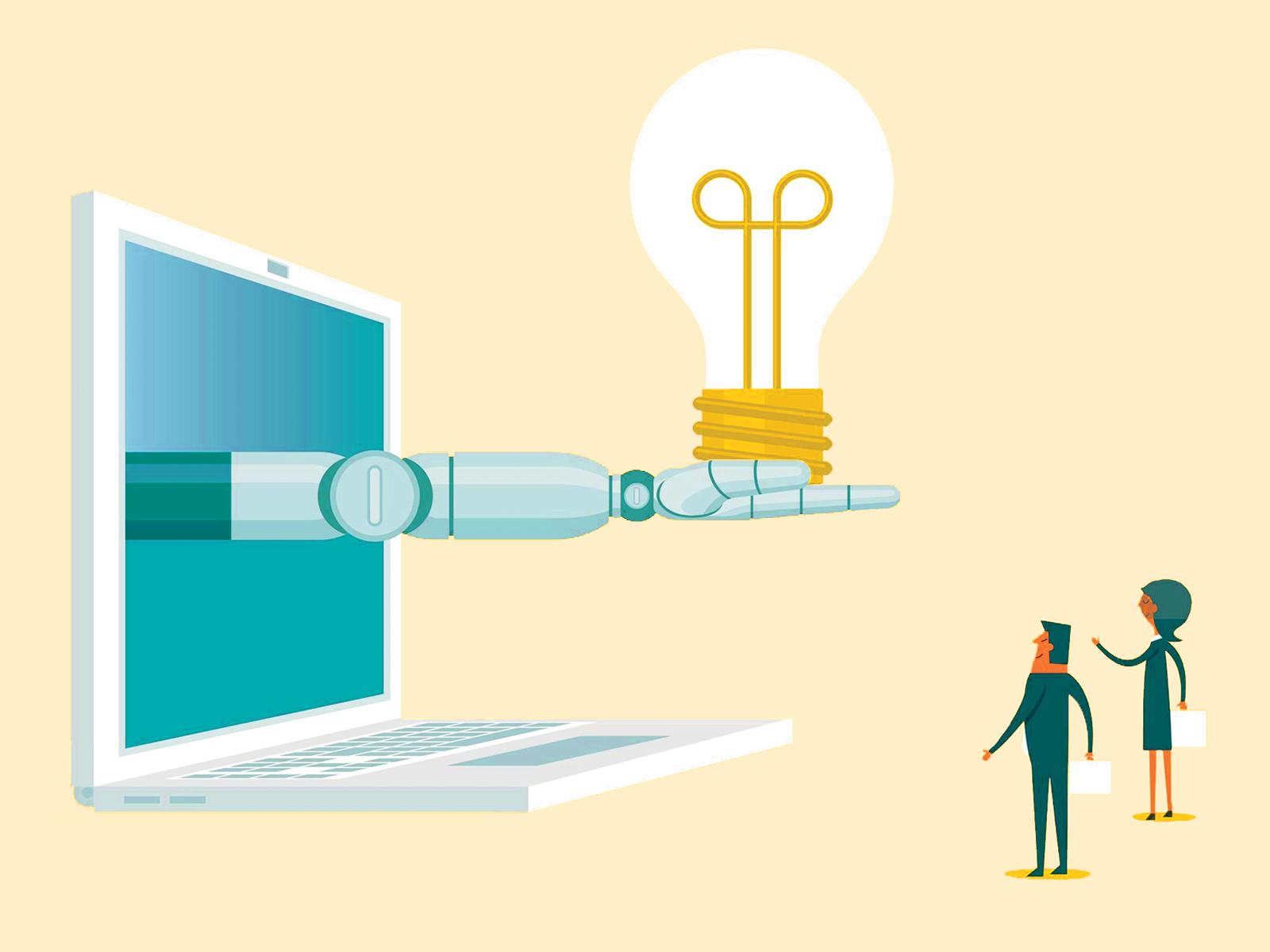
The ethical implications of this are numerous: on the positive side, increased productivity and potential for generating new ideas which can stimulate scientific progress; on the negative side, style theft and impersonation as well as multiple other potential misuses. To take just one example, we used for our training data only articles on which we were first authors and which we had thus largely written ourselves—but nothing prevents others from including whatever articles they want, whether written by themselves or not, in their custom dataset.
In another recent paper, we extended this idea of personalisation to a rather different arena: that of preference predictions for incapacitated patients.4 When individuals are not medically competent to make treatment decisions for themselves—for example, because they are in a coma—others must make these decisions for them, ideally in the same way that the incapacitated person would have made them had they been able to. Unfortunately, it turns out that such ‘surrogate’ decision-makers are little more accurate than chance in these predictions. Moreover, they suffer significant distress and anxiety due to having the burden of decision-making placed squarely on their shoulders.
To counter these problems, ethicists have previously suggested the use of a patient preference predictor: a statistical model which, based on large-scale survey responses by representative samples, could be used to suggest, based on a patient’s demographics, what “others like them” would choose. This information could then be used by a surrogate decision-maker to make their task less burdensome. However, critics have contended that such an algorithm would not, in the relevant ways, respect the incapacitated person’s autonomy, because it would be based on group- and demographic-level data.
In response, we have proposed a personalised patient preference predictor (‘P4’): an LLM fine-tuned, with actual or proxy consent, on an individual’s writings and other information about them (e.g. social media posts, electronic health record information, data from medical interviews and fitness trackers, etc.). We argue that such a P4 would likely be more accurate than both surrogates and the demographic-level algorithm, since it takes into account more relevant information and does so in a more technically rigorous way. But crucially, the P4 would also base its predictions on individual-level information, thus aligning much more closely with the incapacitated individual’s actual desires and preferences (and therefore better respecting their autonomy).
Co-Creation
What should we think of such uses of generative AI—personalised or otherwise? In the scientific arena, the logic of the process implies two different answers: on the one hand, anything that facilitates scientific progress is to be applauded and used, as we need more of this vital substance. This refers to what we might call the outcome goals of science and academia more broadly:5 new knowledge, new cures, new technologies. But academia is also a human battleground in which opposing forces vie for limited positions, funding, and accolades. Seen from this perspective, questions of fairness, disparities, and advantages loom much larger: even if, for the purposes of argument, we assume that personalised (and general) LLMs can facilitate the progress of science, we might also want the process by which these outcomes are realised to be fair, out of respect for the many individuals engaged in these endeavours.
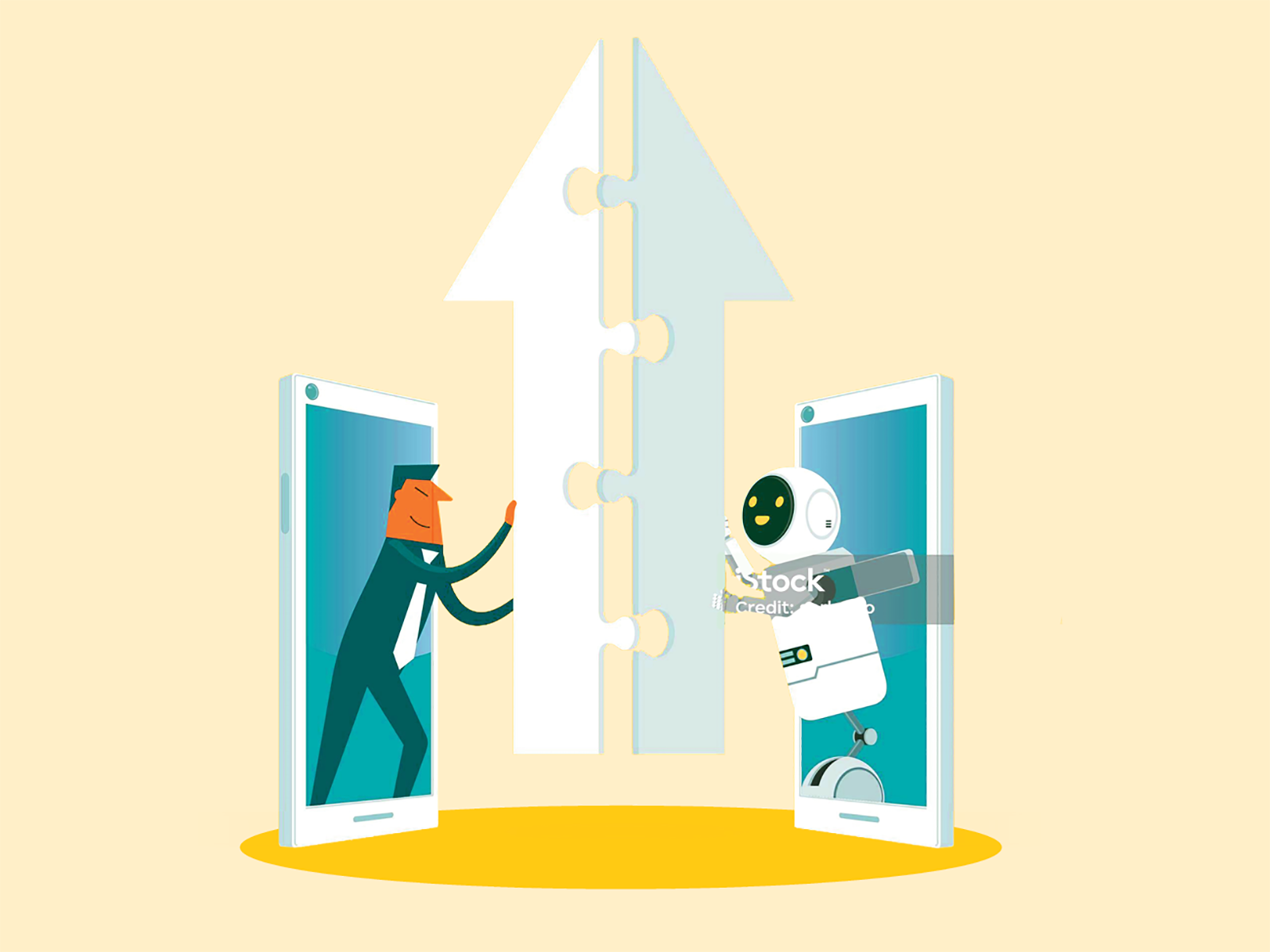
In ongoing work, we sketch a response to these concerns based on the concept of co-creation: while uncritical use (e.g. simple copy-pasting) of LLMs is problematic from the view of both outcome and process, involving essentially an instrumentalisation and servitude on behalf of the human user of LLMs, a more critical and involved process of co-creation is preferable on both counts. In this process, the LLM user does not simply uncritically accept and feed forward the results of an interaction with a language model, but rather serves as a discriminating senior co-author or editor. Under co-creation, the results of an LLM query are meticulously scrutinised for accuracy and fit and are iteratively refined in a process to which both human and machine contribute their special capacities in greatest feasible measure. This idea is aimed at harvesting the potential benefits of LLMs while reducing or avoiding their pitfalls, all the while maintaining, as much as possible, traditional standards for credit and blame attribution.
Conclusion
LLMs are a scientific advance, a general-purpose technology, of the first order. They have the potential to undermine society through misinformation and unintended consequences. But they also have tremendous potential to facilitate science and academia as well as many other areas of life. To maximise their benefits and minimise their harms, difficult conversations need to be had about acceptable uses of these technologies. Our work as described here represent some first tentative steps in that direction.
Porsdam Mann, S., Earp, B.D., Nyholm, S … Savulescu, J. Generative AI entails a credit–blame asymmetry. Nat Mach Intell 5, 472–475 (2023). https://doi.org/10.1038/s42256-023-00653-1.
Maslen, H., Savulescu, J. & Hunt, C. Australas. J. Philos. 98, 304–318 (2019).
Sebastian Porsdam Mann, Brian D. Earp, Nikolaj Møller, Suren Vynn & Julian Savulescu (2023) AUTOGEN: A Personalized Large Language Model for Academic Enhancement—Ethics and Proof of Principle, The American Journal of Bioethics, DOI: 10.1080/15265161.2023.2233356.
Brian D Earp, Sebastian Porsdam Mann, Jemima Allen, … Julian Savulescu. A Personalized Patient Preference Predictor for Substituted Judgments in Healthcare: Technically Feasible and Ethically Desirable. Pre-print, available at: https://www.researchgate.net/profile/Brian-Earp-2/publication/372986750_A_Personalized_Patient_Preference_Predictor_for_Substituted_Judgments_in_Healthcare_Technically_Feasible_and_Ethically_Desirable/links/64d33687b684851d3d92e5ba/A-Personalized-Patient-Preference-Predictor-for-Substituted-Judgments-in-Healthcare-Technically-Feasible-and-Ethically-Desirable.pdf.
Sebastian Porsdam Mann, Pablo de Lora Deltoro, Thomas Cochrane & Christine Mitchell (2018) Is the use of modafinil, a pharmacological cognitive enhancer, cheating?, Ethics and Education,13:2, 251-267, DOI: 10.1080/17449642.2018.1443050.
More from this issue
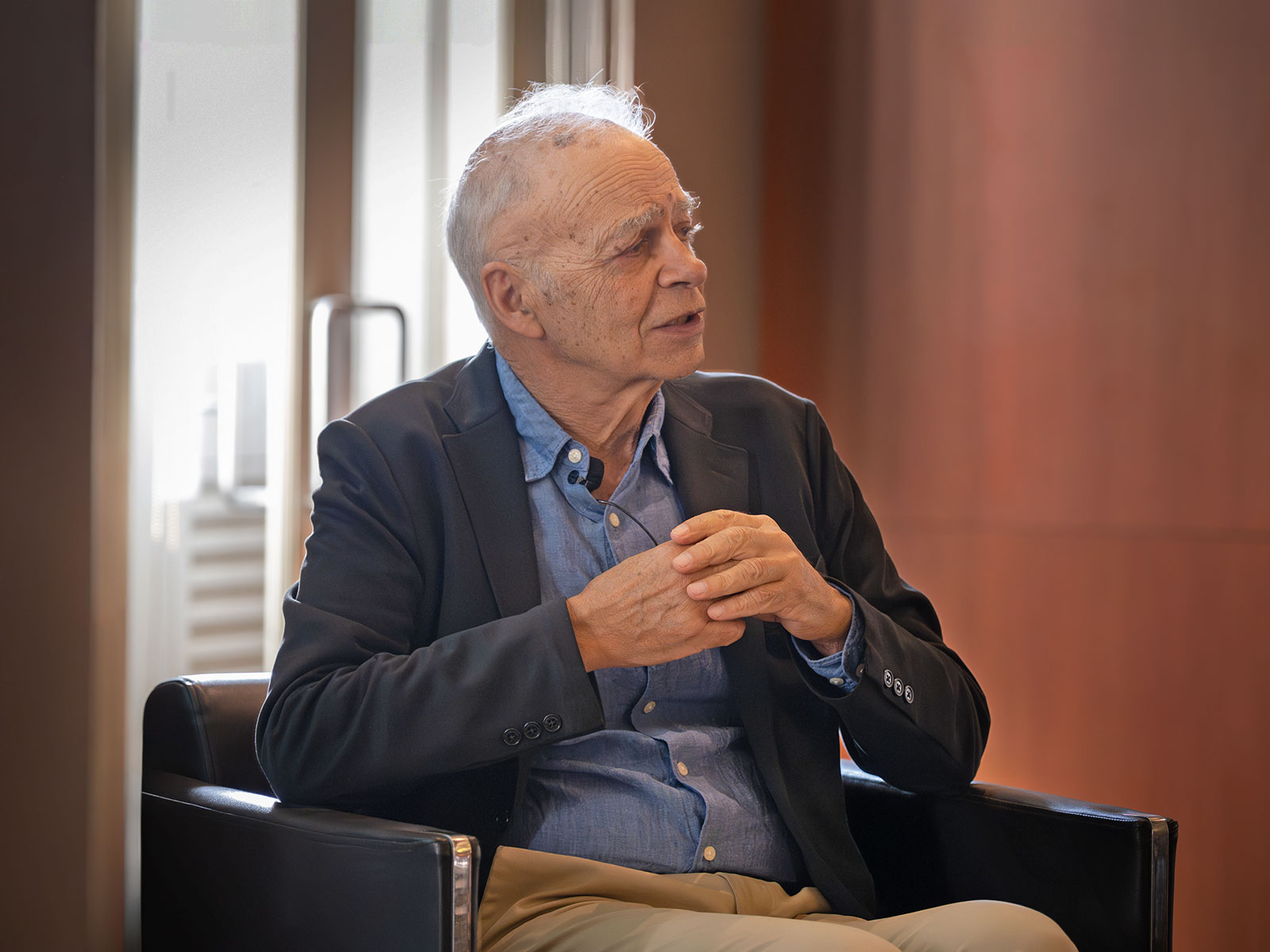
ETHICALLY SPEAKING
Five Takeaways from the Pandemic
AFFAIRS OF THE HEART
Nipping Cardiovascular Disease in the Bud
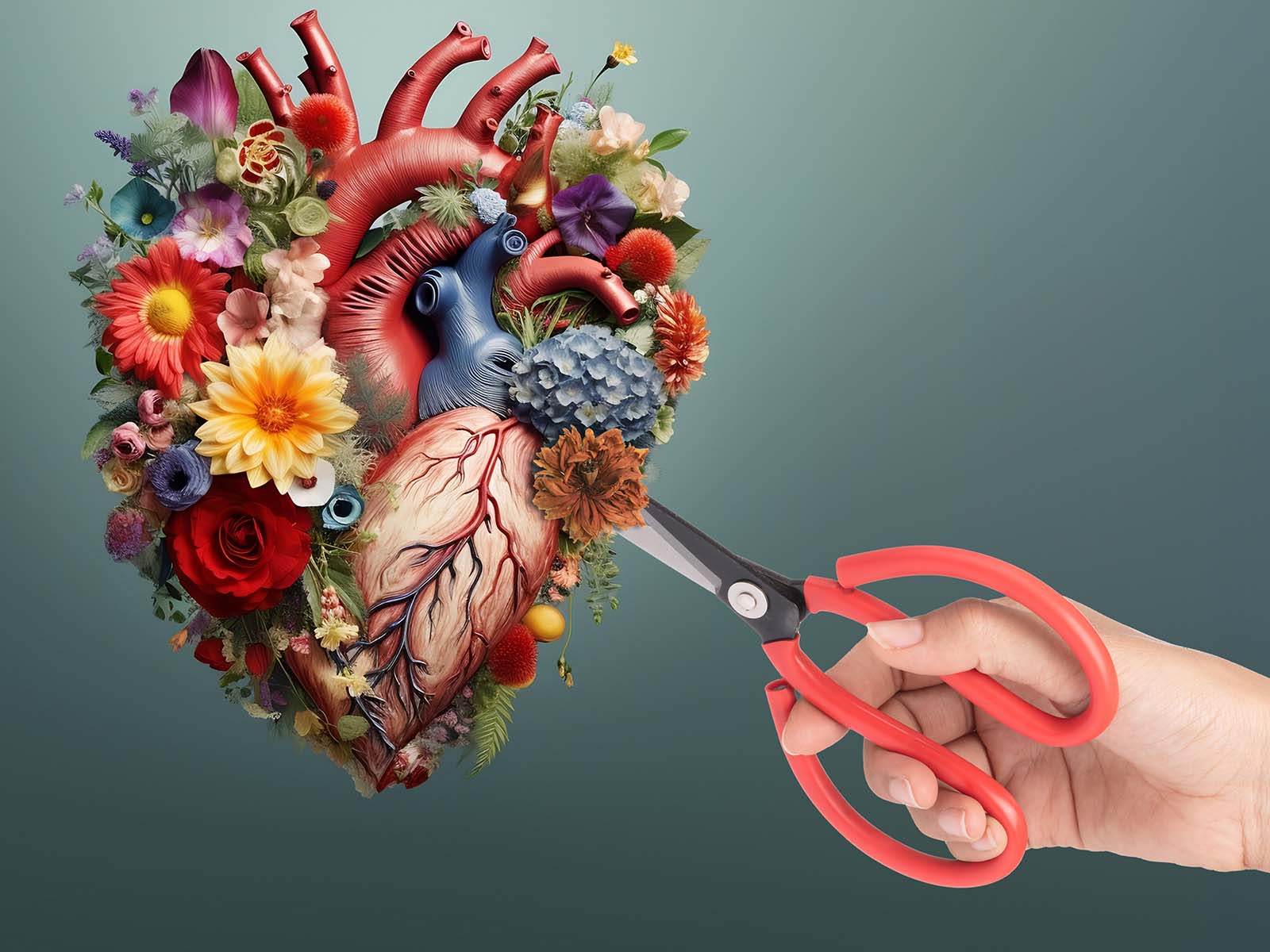
A WORLD IN A GRAIN OF SAND
Antibodies, The Magic Bullets (Zauberkugel) in Medicine
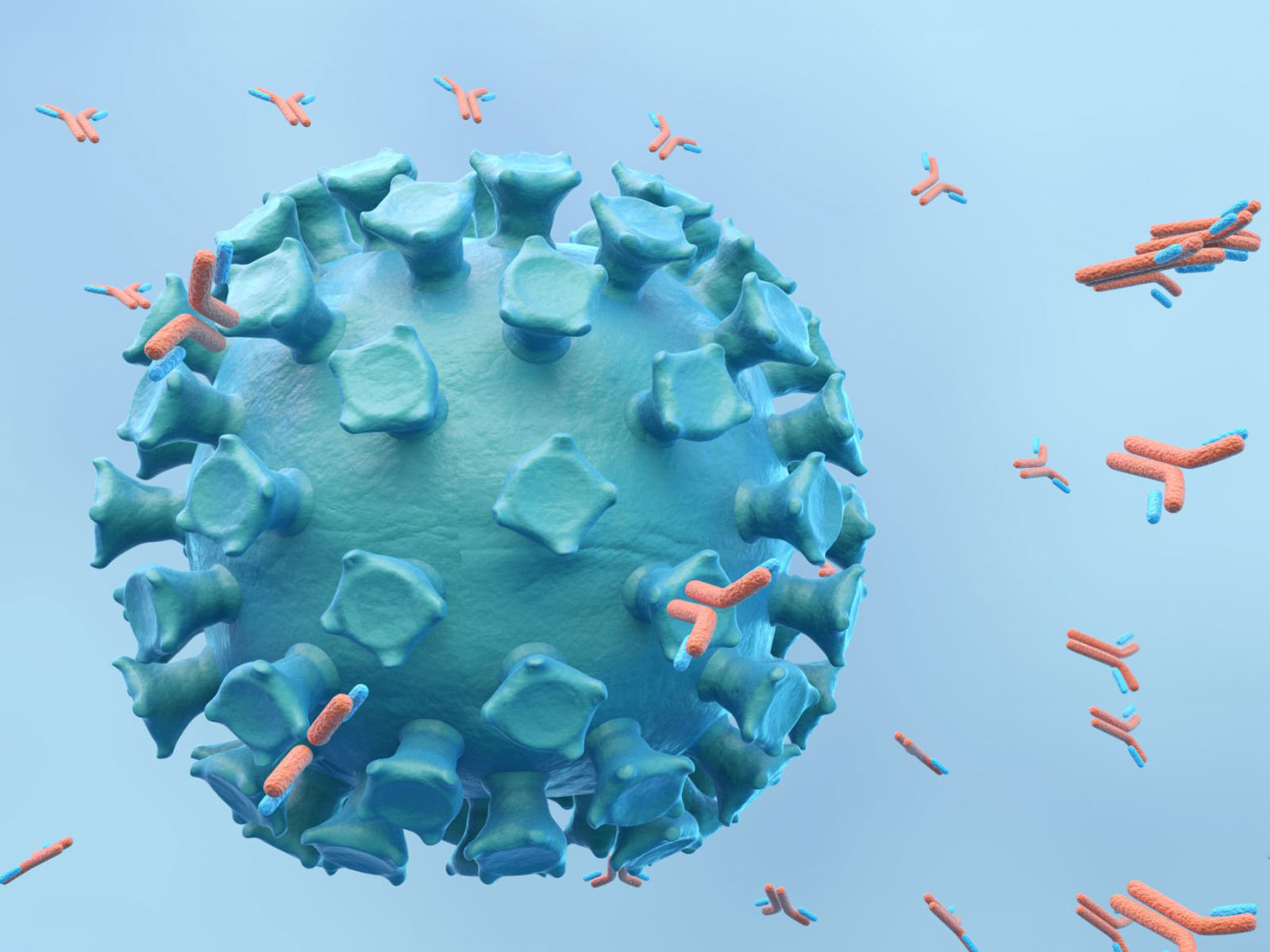