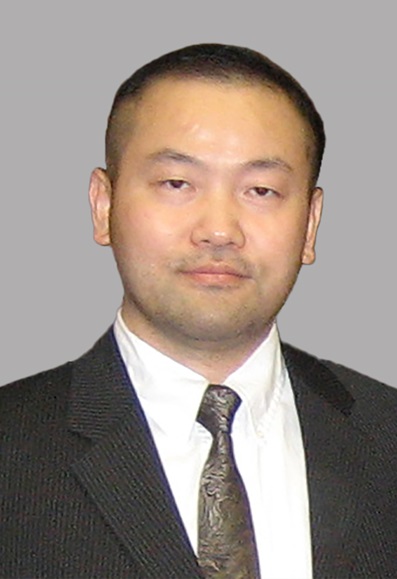
Affiliations
Professor, Department of Biochemistry, Yong Loo Lin School of Medicine, National University of Singapore
Professor, Department of Computer Science, School of Computing, National University of Singapore
Senior Principal Investigator, Cancer Science Institute of Singapore
Biodata
Dr Yang Zhang is a Professor in the Department of Biochemistry at the Yong Loo Lin School of Medicine, National University of Singapore (NUS). He also serves as a Professor and Senior PI in the Department of Computer Science at the School of Computing, and the Cancer Science Institute of Singapore, NUS.
Prior to joining NUS, Dr Yang Zhang worked as a Professor in the Department of Computational Medicine & Bioinformatics, the Department of Biological Chemistry, and the Department of Macromolecular Science & Engineering, University of Michigan. The research interests of the Zhang Lab are in artificial intelligence (AI) and deep neural network learning, protein and RNA structure prediction, and protein design and engineering. The I-TASSER algorithm (https://zhanggroup.org/I-TASSER/) developed in his laboratory was ranked as the No 1 most accurate method for automated protein structure prediction in the community-wide CASP experiments nine times in a row since 2006. The Zhang Lab is one of the first laboratories who initiated the studies on deep machine-learning based protein and RNA structure predictions, while the artificial intelligence technique has brought about revolutionary impact on many fields in life science. Among the honours that Dr Zhang received includes the Alfred P Sloan Award, the US National Science Foundation Career Award, and the University of Michigan Basic Science Research Award. He was selected as the Thomson Reuters/Clarivate Analytics Highly Cited Researcher for six times since 2015.
Research Interest
- Artificial intelligence and deep neural network learning
- Protein folding and structure prediction
- Protein/peptide design and drug discovery
- RNA design and RNA structure prediction
Current Research Projects
AI-based protein and RNA structure prediction. Proteins are the “workhorse” molecules of life and what work proteins do in cells depend on their 3D structure. Protein structure prediction, which aims to determine the spatial location of every atom in proteins from the amino acid sequence by computational simulations, represents one of the most important problems in computational biology. Historically, the field of protein structure prediction has been dominated by comparative modeling which relies on the availability and detection of homologous templates from the Protein Data Bank (https://pubmed.ncbi.nlm.nih.gov/18436442/). Our lab is one of the first laboratories who initiated the studies on deep learning based protein and RNA structure predictions, which have dramatically changed the scope and accuracy of computer-based modeling of biomolecular structures (https://www.nature.com/articles/d41586-021-03499-y). In this project, we are developing new algorithms by integrating cutting-edge AI techniques with the fragment assembly simulations (I-TASSER, https://zhanggroup.org/I-TASSER/) for large-size proteins and protein-protein complex structure determinations. We are also extending the AI methods for high-quality structure modeling of RNAs which have been increasingly recognized as with important biological function and drug design potential including vaccines, in addition to their canonical roles in transcription and translation.
AI-based protein design and drug discovery. Proteins in nature were created following billions of years of evolution and therefore possess limited structural folds and biological functions. Computational protein design aims to design and engineer new protein and peptide sequences with novel structure and function beyond nature proteins. Given their potential to create new functions and change cellular pathways, computationally designed proteins and peptides can be used as drugs to treat pharmaceutically important human diseases such as cancer and Alzhelmer’s disease. In this project, we are developing new AI-based approaches for high-accuracy de novo protein and peptide design by the integration of protein language model and transformer networks with physical and evolutionary profile simulations (e.g., EvoDesign, https://zhanggroup.org/EvoDesign/). We are particularly interested in the application of the AI-based designing algorithms for effective cancer therapeutics and treatment, with focuses on anti-cancer peptide-drug conjugates (PDCs), T-cell receptor (TCR)-based adoptive therapy, and antibody discovery and optimization.
Selected Publications
(A full list of Dr. Yang Zhang’s publications can be seen at https://scholar.google.com/citations?user=MtBs-kMAAAAJ)
- Zheng W, Wuyun Q, Li Y, Zhang C, Freddolino PL, Zhang Y. Improving deep learning protein monomer and complex structure prediction using DeepMSA2 with huge metagenomics data. Nature Methods, in press (2023).
- Li Y, Zhang C, Feng C, Pearce R, Freddolino PL, Zhang Y. Integrating end-to-end learning with deep geometrical potentials for ab initio RNA structure prediction. Nature Communications, 14: 5745 (2023).
- Pearce R, Huang X, Omenn GS, Zhang Y. De novo protein fold design through sequence-independent fragment assembly simulations. PNAS, 120: e2208275120 (2023).
- Zhang C, Shine M, Pyle AM, Zhang Y. US-align: Universal Structure Alignment of Proteins, Nucleic Acids and Macromolecular Complexes. Nature Methods, 19: 195-204 (2022).
- Zhou X, Zheng W, Li Y, Pearce R, Zhang C, Bell EW, Zhang G, Zhang Y. I-TASSER-MTD: A deep-learning based platform for multi-domain protein structure and function prediction. Nature Protocols, 17: 2326-2353 (2022).
- Zhou X, Li Y, Zhang C, Zheng W, Zhang G, Zhang Y. Progressive assembly of multi-domain protein structures from cryo-EM density maps. Nature Computational Science, 2: 265-275 (2022).
- Zhang X, Zhang B, Freddolino PL, Zhang Y. CR-I-TASSER: assemble protein structures from cryo-EM density maps using deep convolutional neural networks. Nature Methods, 19: 195-204 (2022).
- Mortuza SM, Zheng W, Zhang C, Li Y, Pearce R, Zhang Y. Improving fragment-based ab initio protein structure assembly using low-accuracy contact-map predictions. Nature Communications, 12: 5011 (2021).
- Yang P, Zheng W, Ning K, Zhang Y. Decoding the link of microbiome niches with homologous sequences enables accurately targeted protein structure prediction. PNAS, 118: e2110828118 (2021).
- Zheng W, Zhang C, Li Y, Pearce R, Bell EW, Zhang Y. Folding non-homology proteins by coupling deep-learning contact maps with I-TASSER assembly simulations. Cell Reports Methods, 1: 100014 (2021).